Tado
Rare are the artists that dare to innovate; Tado is one of them.Tado, or Will Furtado, is an independent musician and lyricist hailing from Berkeley, California. Growing up in a small coastal region of Massachusetts, Tado cites his brief stint with the Dem Roots rap crew to have catalyzed his passion for music. Although the majority of his discography remains unreleased, the most zealous fans are known to have access to recordings from his early career.Lauded by many, Tado's highly-anticipated debut release, Scooby, was a collaborative project with his former roommate and friend, Jinho Shin. Superficially, the track details unrequited love; on a more profound level, it raises the moral dilemma of choosing between what one is truly passionate about, and what one knows will give them economic success.With a renewed lust for artistry, Tado made his return on the eve of his 21st birthday with his sophomore single, Telegraph, this time partnering with Shin under a new moniker: Sappho.Rounding out the triumvirate of pop classics, 510 features Tado flaunting nimble wordplay and a pugnacious flow.
About me
My name is Will and I'm a Machine Learning Engineer at SwingVision.I grew up in Cape Cod, Massachusetts and received my International Baccalaureate Bilingual Diploma from Sturgis Charter School in 2019. I then went on to attend UC Berkeley where I graduated with degrees in Applied Mathematics and Computer Science.Recently, I led the ML development and release of SwingVision’s electronic line calling system. I revamped model architectures, improving "close call" accuracy from 93% to 97%, which led to widespread adoption at junior and college tournaments. The system is now used by thousands of players at official USTA tournaments) across the country.At Cambridge Mobile Telematics, I designed and built a Python module to process vehicle telemetry data, run statistical inference tests, and automatically generate device reports for customers. This tool reduced report creation times from ~2 weeks to under 10 minutes and was a key component in securing $5M+ deals with enterprise customers.
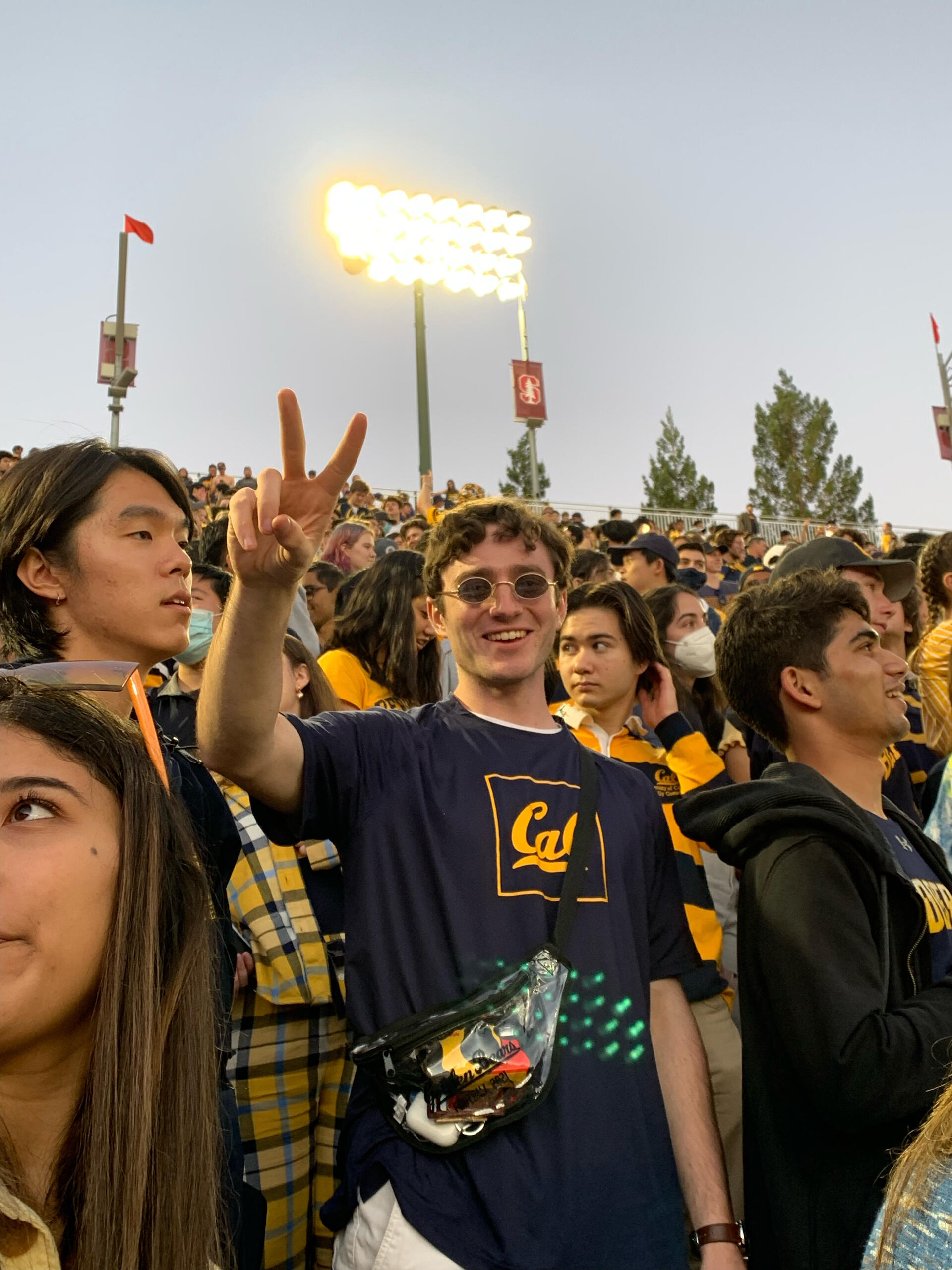
Academics
Growing up it was always math that drove my intellectual curiosity. As such, I came to Berkeley solely intending to major in Applied Mathematics. I had never written a line of code until I took Data 8 my freshmen fall. That course, which I taught for several years, is what catalyzed my love for computing; it was spectacular how many doors were opened after I learned to harness the power of code.After taking the lower division CS courses, I decided to pursue a double major in Applied Math and Computer Science. Nevertheless, my love for mathematics only grew fonder after I saw how entangled the two fields were. Sheldon Axler's Linear Algebra Done Right proved infinitely valuably in my later coursework, particularly in Malik's offering of CS189.As I continued to engulf my math and computer science courses, something felt missing. Unsurprisingly, that something was data science. Per Professor Adhikari's recommendation, I enrolled in Data 140 -- a class which I encourage everyone to take -- and Data 188, the pilot offering of a statistical inference seminar. The latter taught me that conclusions are only as valid as the assumptions upon which they rest.
As of Late
When not shooting film through the lens of my Nikon F3, you'll likely find me curating my Spotify playlists, scouring Beli for the best Neapolitan pizza, or writing pop songs with far away friend Jinho Shin.Regardless of the weather, I love to play tennis or a cheeky bit of footy. Come winter time, the slopes becomes my top priority; the strongest of recommendations for Corbet's Couloir in Jackson Hole, Wyoming. Soon, I hope to conquer the Swiss Alps via heli-skiing.I find relaxation in los versos sencillos of José Martà and Neruda's Poema 20. Their work inspired me to persevere in my quest of Spanish fluency, so much so that I began to dabble with the quill through lines of my own.Top Three Movies: (in no particular order) Good Will Hunting, 10 Things I Hate About You, SuperbadAnd there's that one time an unforgiving tree broke my spine, but I'll save that for another day.
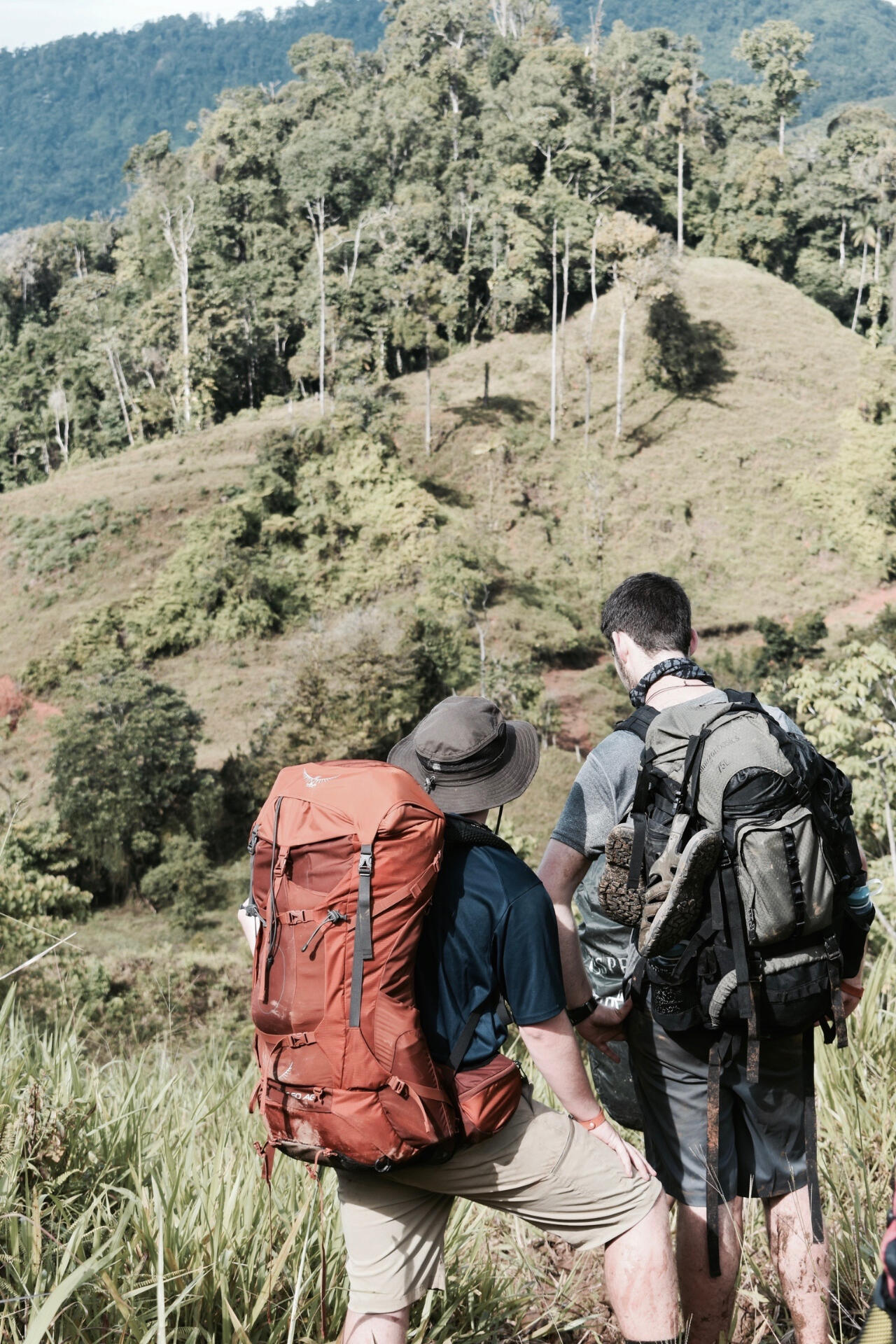
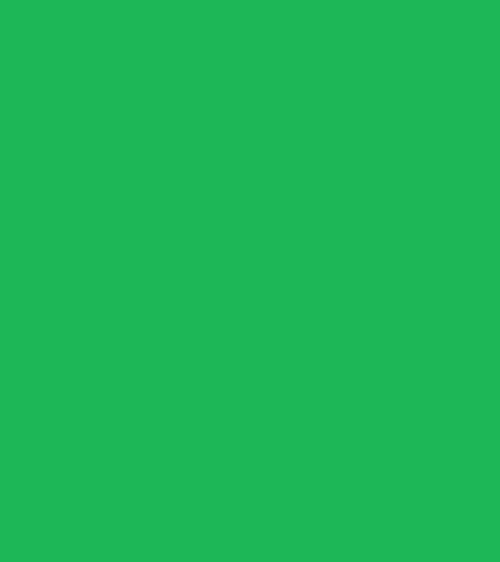
Triple Threat
Over the past several years, my love for the Spotify platform has grown unconditionally. As a direct result of this obsession, I have become what I will call the Spotify Triple Threat.
Avid Consumer: Raking in well over 250,000 listening minutes on Spotify, it is clear that I know the brand from a consumer's perspective inside and out.
Spotify Developer: Working with Spotify's Web API and the Spotify for Developers platform has allowed me to understand what working backstage is truly like.
Verified Artist: Familiarity with Spotify for Artists and a heightened awareness of the music distribution process prove invaluable when working on features used by top artists today.
Projects
Course Projects
Augmented Reality (Python, OpenCV)
cs194-26
Image Morphing (Python)
cs194-26
Pac-Man AI (Python)
cs188
Housing Appraisal GLM (Pandas)
data100
Spam Filter (scikit-learn)
data100
Deque API (Java)
61b
Code available upon request for course projects.
Independent Projects
Coursework
Mathematics
Computer Science
Data Science
Please don't hesitate to reach out to me about anything! I'll take any chance I can get to fuel my caffeine addiction.
Cheers
Once I see your message, I'll drop everything to get back to you as quickly as I can.Please don't hold me to that.
The Triumvirate of Spotify Playlists
Forget this website, get to know me through my music taste. Here are three of my favorite playlists at this moment in time.
Photo Archive
Curated for my own enjoyment, available for all to view. I'd called this a fair, albeit limited, representation of myself.
Teaching
Appointments
Foundations of Data Science: Course Director for the Fall 2022 and Spring 2023 offerings of Data 8; Lead Student Instructor (Pedagogy) for Fall 2021 and Spring 2022; Small Group Tutor during Spring 2021.
Introduction to Computational Thinking with Data: Co-Instructor for the Summer 2022 offering of Data 6; Responsible for curriculum development for future semesters.
Berkeley Unboxing Data Science (BUDS) Program: Associate Program Facilitator for the Summer 2021 offering of the BUDS high school program.
Awards
Outstanding Graduate Student Instructor (OGSI): Awarded by the College of Computing, Data Science, and Society for outstanding work in the teaching of undergraduates.
Materials
Media
"The Place To Be": A Look Into Data Science at UC Berkeley - The Daily Californian
Berkeley Unboxing Data Science Program Doubles Number of Interns in Second Year - Berkeley CDSS